The transport sector is one of the main sources of CO2 emissions. Along with increasing the share of e-vehicles, rail transport is intended to help achieve the European climate protection targets (reduction of CO2 emissions) in the transport sector. Consistent digitalization and automation of train operations is an important part of a climate-neutral and attractive transport mix. In particular, driverless trains play an important role in increasing the attractiveness of rail transport by offering a broad and flexible range of services. Specifically, this requires a higher frequency of train service (shorter cycle times), better reliability (higher punctuality) and greater flexibility in operational processes. In addition, driverless trains can help to counteract the shortage of train drivers and reduce operating costs (energy costs as well as maintenance costs) through optimized operation.
About the project
How do we imagine the future of mobility?
Project consortium
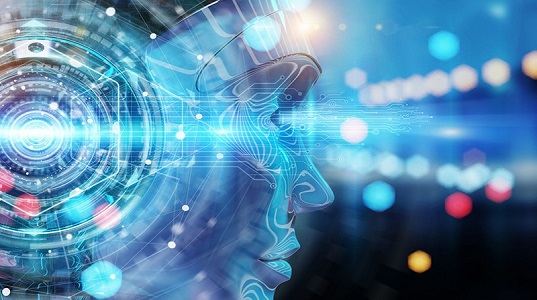
Our vision explained in a few words.
In a safety-critical environment such as driverless rail transport, AI methods are needed that are demonstrably robust and safe, for example, in order to detect obstacles on the track with the necessary reliability. The safe.trAIn project aims to lay the foundations for the safe use of AI for driverless rail vehicles and thus address the greatest technological challenge for the introduction of driverless regional transport.
The advantages of AI-controlled regional trains
Driving automation in regional rail transport
In a consortium of technology suppliers, research institutions, and standardization and testing organizations, our experts are working on combining the possibilities of artificial intelligence with the safety considerations of rail transport. Based on the requirements for safety verification, test methods and tools for AI-based methods are being developed for use in a driverless regional train and then validated and verified in a virtual test field. The focus here is on AI-based functions for object recognition.
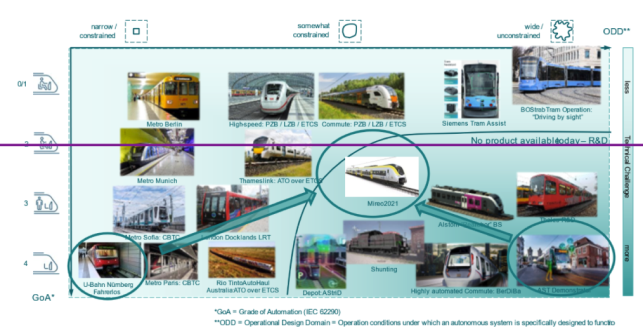
The following figure shows the current situation of the different automation levels GoA 1-4 and their application under different public transport operating conditions (narrow to wide).
Project organization
The project has been structured in six work packages. These are interconnected and mutually dependent. The first phase of the project is definition of the requirements for the safety case procedure. Suitable methods and tools as well as a safety architecture are identified for this purpose.
A virtual test environment is necessary for the safety analysis. The results will be translated into standardization activities during the project and can be communicated for further exploitation.
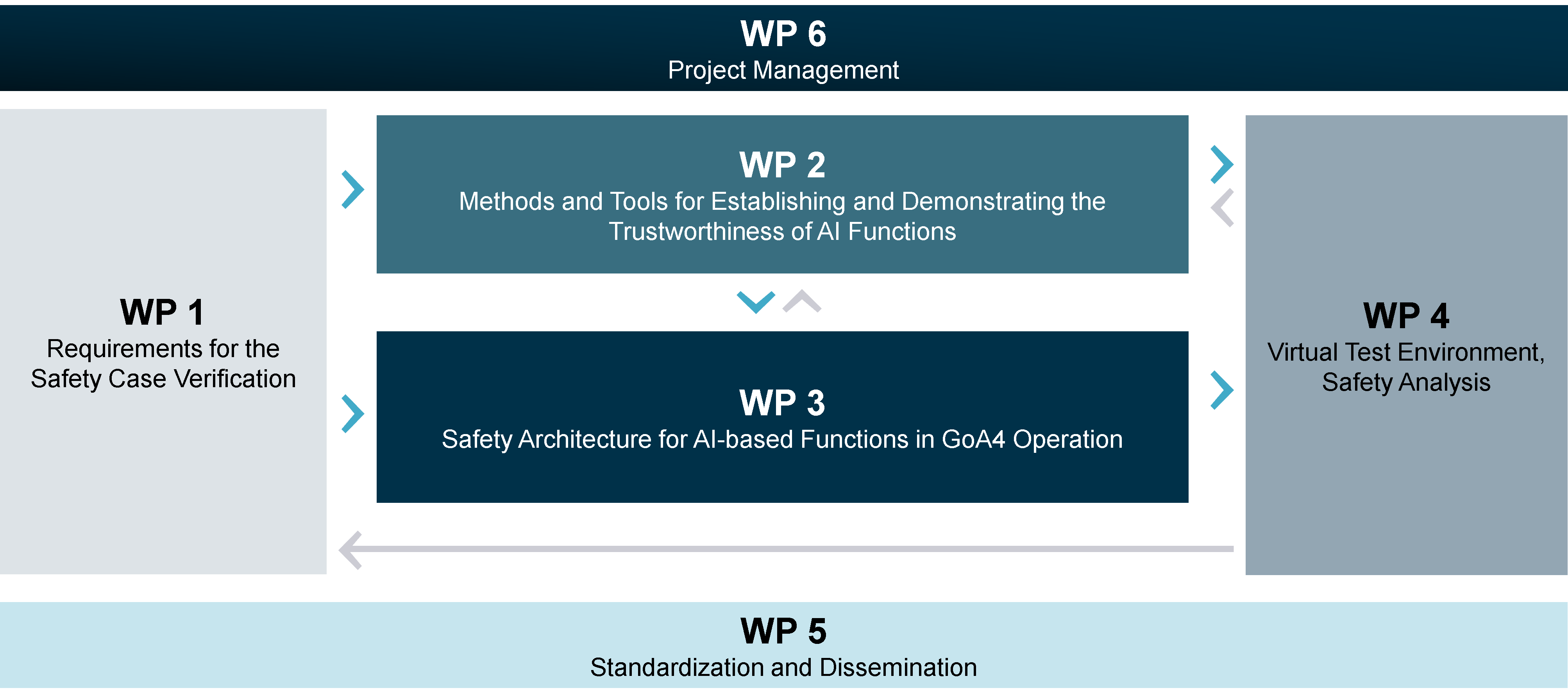
WP1: Requirements for the Safety Case Verification
The purpose of the work package is to research and survey the existing standards in terms of applicability to AI-based and ML-based safety functions, derivable requirements for and properties of such safety functions, and the methods, tools and metrics to be used.
As a result, this work package will provide requirements and guidelines for demonstrating the product safety of a GoA4 rail vehicle with AI-based safety-related functionalities for certification purposes.
To this end, the work package is subdivided into the following topic areas:
Applicability analysis of existing standards
All existing standards and norms from the areas of rail, industry, automotive, security, functional safety and AI in general will be recorded and examined in order to ascertain the extent to which they can also be applied to new, safety-critical AI systems.
The focus here is on researching relevant activities and existing norms, standards, harmonized norms under the NLF and CSA schemes, as well as best practices and experiences with relevant AI applications. The final step will be to derive the applicability and the limits of existing norms and standards, for example from the abovementioned areas.
Derivation of properties and acceptance criteria to be demonstrated for the safety functions
The basic properties of and requirements to be met by AI-based systems for automatic train control must be suitable for ensuring a level of safety which is equivalent to the classic approach for assessing systematic faults (e.g. Safety Integrity Levels according to IEC 61508/EN 50129).
The derived properties and acceptance criteria to be demonstrated will form the basis for the subsequent verification and validation of the safety of the AI-based application for automatic train control.
Requirements to be met by methods, tools, development processes and operation
On the one hand, requirements will be drawn up for methods and tools for the verification, validation, testing and assessment of AI systems. In the specific use case, the focus here is on perceptive AI applications based on neural networks. On the other hand, requirements will be determined for processes for developing and operating AI systems.
Quantifiable metrics for assessing the trustworthiness, declarability and safety performance of AI
Based on the previously defined properties and acceptance criteria to be demonstrated and the results of the applicability analyses of existing norms and standards, state-of-the-art metrics will be researched and analyzed in order to provide a quantitative assessment of system-side safety objectives and AI-specific properties.
Here, the focus is on defining requirements and metrics in order to be able to assess objectively whether the acceptance criteria for the certification of an AI function are met in the proposed use case.
WP2 Methods and Tools for Establishing and Demonstrating the Trustworthiness of AI Functions
The objective of this work package encompasses two central topic areas. The first relates to the systematic creation of safety requirements for the AI-based systems used within the project. The second area focuses on the creation of methods and proofs that can be used to validate the AI requirements defined in the first area.
Considered in more detail, the two areas of the work package comprise the following points:
Demonstration of the trustworthiness of the AI systems
Based on the properties and acceptance criteria to be demonstrated as defined in WP1, a demonstration methodology and all safety-related requirements for the AI systems will be drawn up. The aim is to demonstrate with sufficient confidence that these requirements are met so that the residual risk relating to a malfunctioning of the AI-based system is accepted as tolerable. In addition, the effectiveness of the proposed proofs for validating the demonstration strategy will be investigated. At the end of the project, there will also be a review of the safety case as a whole.
Methods and tools for assessing the trustworthiness of the AI systems
Based on the defined safety-related requirements to be met by the AI systems, metrics, methods and tools will be developed as proofs to support the proposed demonstration. These proofs comprise the following aspects of the safety case of an AI system:
- The description of the operating environment and assurance of data quality
- Methods and architectures for integrating AI applications with classic approaches
- Methods for checking the robustness of the AI systems in order to minimize the uncertainties inherent in the model
- Methods for checking the reliability and transparent behavior of the AI systems
- Methods for verifying the intended behavior of the AI functions
- Methods for monitoring and increasing reliability and for excluding faults during runtime
WP3: Safety Architecture for AI-based Functions in GoA4 Operation
This work package covers the development of an integrated basis for requirements and architecture as well as overall system implementation (system under test) and the safety case for the AI-based object detection of a driverless regional train.
The work package is subdivided into 4 topic areas:
Requirements
Based on results from WP1 and findings from research projects at the national level (e.g. ATO-Risk, ATO-Sense) and at the European level (e.g. X2Rail, TAURO), the requirements to be met by a driverless regional train with an AI-based object detection system will be collected, prioritized, and integrated into an overall structure. This will be supplemented by the definition of the operational design domain, in which the environmental conditions (operational, climatic and weather-related constraints), including the persons, obstacles and systems interacting with the system, are recorded.
Architecture
Based on the defined requirements, an architecture for a "driverless regional train" will be drawn up for the object detection system, the sensor fusion and the sensor system, in which the interfaces between the functions are defined at all 4 architecture levels and the RAMS requirements (reliability, availability, maintainability and safety) as well as NFRs (non-functional requirements) are taken into consideration.
Implementation
In the third subarea of work package 3, the overall system will be implemented and integrated as a software solution. The ML functions/models provided for in the architecture will be implemented here. These form the basis for the methods and metrics developed in WP2 for investigating the trustworthiness and validation. In addition, a hardware demonstrator will be developed and tested for the sensor system.
Safety case procedure
The final subarea covers the development of a concept for a safety case for a driverless regional train with an AI-based object detection system, combining the results of the upstream activities in the area of requirements, architecture and metrics. The structure of the safety case serves as the basis for evaluation in the virtual test environment (WP4) and subsequent assessment.
WP4: Virtual Test Environment, Safety Analysis
WP4 covers the development of a virtual test environment for evaluating the project objectives in safe.trAIn. In this virtual test environment, the validation of an AI function in the rail transport sector can be carried out using the example of obstacle detection.
The work package is subdivided into the following topic areas:
SafeMLOps
Based on the results of work packages 1-3, an end-to-end development infrastructure for AI methods for rail vehicle applications will be designed and implemented as a prototype into which the investigated methods are integrated. To this end, the test methods required to generate proofs (defined in WP2) for the safety case procedure will be integrated into what is known as a SafeMLOps process and automated.
Virtual test environment
In order to implement this SafeMLOps approach as a prototype, a virtual test environment for driverless vehicles will be developed in WP4 for the purpose of validating the test criteria for AI functions. This virtual test environment will serve as a validation platform, integrating the different test methods developed in WP2 and implemented in WP3 in order to verify the trustworthiness of an AI function and generate proofs for the safety case procedure. Here, the AI function will be validated by means of software-in-the-loop methods with the aim of carrying out comprehensive empirical tests (simulations, etc.) with the AI functions and obtaining metrics for the safety case procedure. These simulations will be carried out with both real and synthetic data.
Evaluation
The results from the virtual test environment will then be compared with the defined test criteria of the certification process and embedded in a safety case for driverless rail vehicles. This will enable an initial set of rules to be tested under real conditions, from assessment through to conformity declaration for the present use case, and will allow conclusions to be drawn as to the applicability of test criteria and test methods in the certification process. The results obtained here will be forwarded continuously to WP1, WP2 and WP3.
WP5: Standardization and Dissemination
Work package 5 deals with the standardization and distribution of relevant results from the safe.trAIn project. This will facilitate a broad application of the results even after the end of the project.
The work package is divided into the following focal topics:
Standardization activities
To ensure the successful transfer of results into standardization activities, various tasks are addressed in the corresponding work package sections. The essential tasks are to conduct an analysis of the standards identified in work package 1 as relevant to the project and to identify where there is a need for standardization, e.g. standardized interfaces, processes, test procedures, etc. These will be transferred to standardization activities in close collaboration with all project partners and relevant standardization committees. The aim is to transfer relevant project results into standardization with the involvement of other stakeholder groups. There is also a focus on ensuring the most strategically effective distribution of relevant project results at the national, European and international standardization levels.
Formation of user groups
Another task is to set up a networking and discussion platform. This will take the form of user groups, with the aim of validating project results with other expert stakeholders, considering use cases and where appropriate identifying joint standardization potential.
Consideration and analysis of the AI ecosystem in terms of normative legislation
In view of the changing legislative framework for normative requirements in the world of artificial intelligence, the transfer of project results (e.g. validation argumentation) to established dimensions for trustworthy AI (e.g. data protection, fairness, transparency, autonomy and control, technical documentation, robustness, reliability, safety, etc.) will be investigated. In addition, a methodology will be developed for the fulfillment of all regulatory requirements, as stipulated for example in the current draft of the European AI regulatory framework. This will be supplemented by an analysis of the project-specific effects of further regulatory developments (e.g. Cyber Resilience Act).
Distribution of results
The project results are also to be published outside the safe.trAIn project. To this end, it will be necessary to define measures in order to ensure that the project activities are communicated effectively, and to support this process.
Project funded by the German Ministry for Economic Affairs and Climate Action.
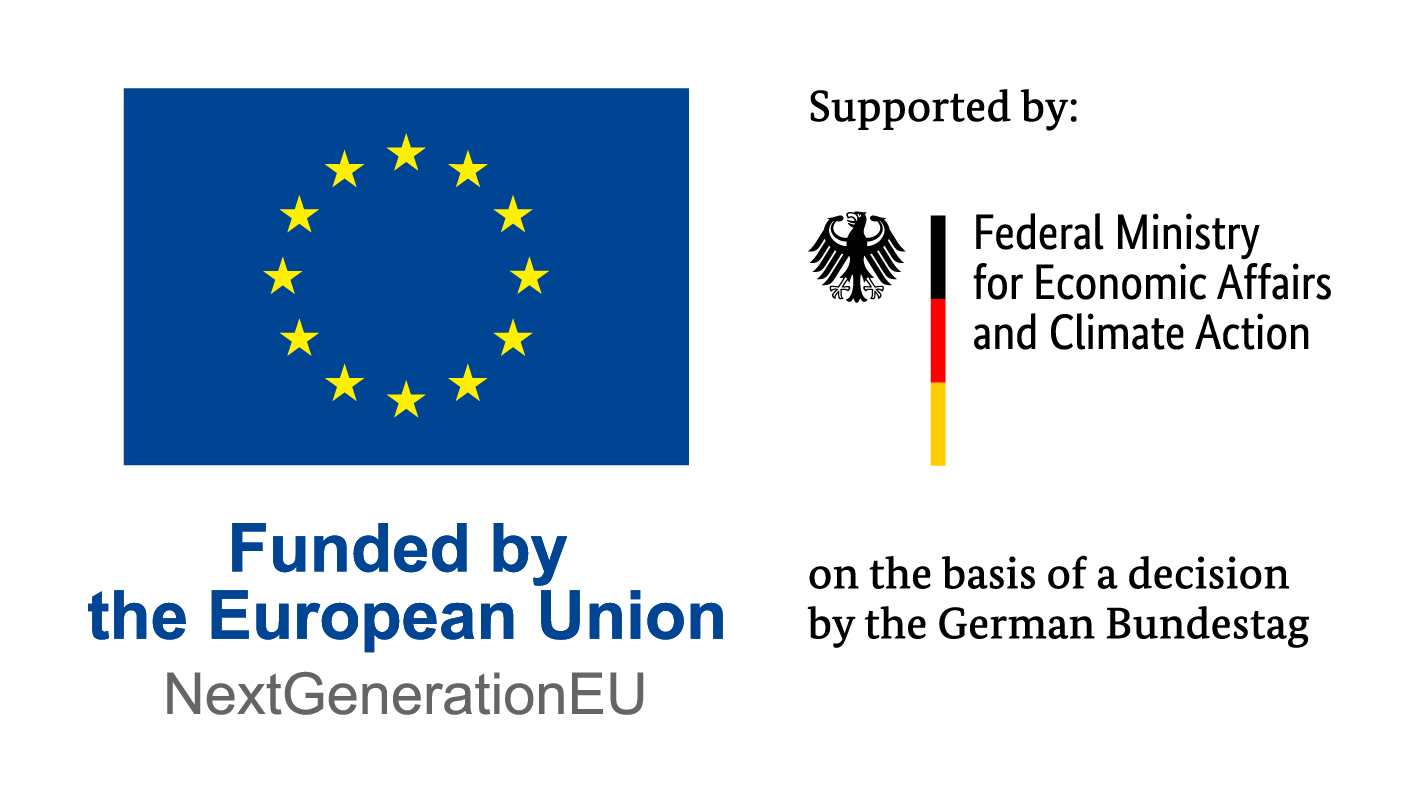
You want to learn more about safe.trAIn? Get in touch!
© Siemens 1996 – 2024